Expand Log20 M N5p – Dive into the power of logarithms!
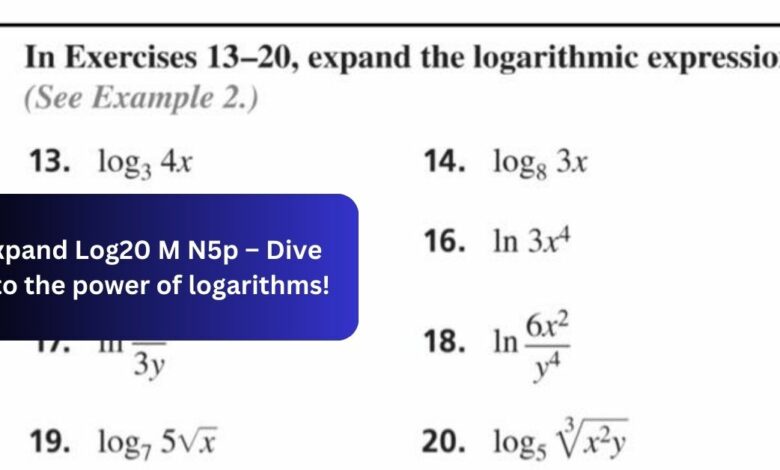
Understanding logarithmic expressions and their expansion is a fundamental aspect of mathematics with wide-reaching applications. In this introductory exploration, we delve into the process of expanding a logarithmic expression of the form log20(mn5p).
To expand the logarithmic expression log20(mn5p), we apply the product rule of logarithms, which states that the logarithm of a product is the sum of the logarithms of the individual terms. Therefore, the expanded form of log20(mn5p) is log20(m) + log20(n) + log20(5p).
Let’s embark on this journey to unlock the secrets behind expanding logarithms.
Explore the Concept – Unlock the Mystery!
A logarithm is a mathematical function that measures the exponent needed to produce a given number. In simpler terms, it tells us what power we need to raise a certain base to in order to obtain a specific value. Logarithms are widely used in various fields such as mathematics, science, engineering, finance, and computer science.
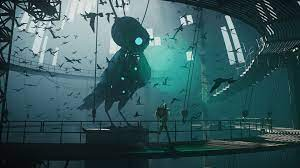
Here’s a more detailed explanation:
Definition: In its basic form, the logarithm of a number x to a given base b is the exponent to which the base must be raised to produce the number x. In mathematical notation, it is denoted as logb x.
Properties of Logarithms:
Product Rule: logb (xy) = logb(x) + logb(y)
Quotient Rule: logb (x/y) = logb(x) – logb (y)
Power Rule: logb (xa) = a . logb(x)
Change of Base Formula: logb (x) = logc (x)/logc (b)
Common Bases: While logarithms can have any positive base, some common bases include 10 (common logarithm), e (natural logarithm), and 2 (binary logarithm).
Exponential Growth and Decay – Unlock the Power of Mathematics!
Exponential growth and decay are fundamental concepts in various fields, including mathematics, economics, biology, and physics. These phenomena describe how quantities change over time, either increasing or decreasing at an accelerating rate.
Exponential Growth:
In exponential growth, a quantity increases at a rate proportional to its current value. This means that as time progresses, the rate of increase also grows. Mathematically, exponential growth is represented by the equation:
N(t)=N0 ×ekt
Where:
- N(t) is the quantity at time t.
- N 0 is the initial quantity (at t=0).
- k is the growth rate constant.
- e is Euler’s number, approximately equal to 2.71828.
Exponential growth is commonly observed in populations, investments, and the spread of infectious diseases.
Exponential Decay:
Conversely, exponential decay describes the decrease of a quantity at a rate proportional to its current value. This means that as time passes, the rate of decrease also accelerates. Mathematically, exponential decay is represented by the equation:
N(t)=N0 ×e−kt
Where the variables have the same meanings as in exponential growth.
Exponential decay is frequently encountered in radioactive decay, the cooling of objects, and the depreciation of assets.
Applications:
Exponential growth and decay models have numerous applications in real-world scenarios. In finance, compound interest is a classic example of exponential growth, where investments grow at an accelerating rate due to the reinvestment of interest.
In epidemiology, exponential growth models help predict the spread of diseases based on factors like infection rates and population density. Similarly, exponential decay models are crucial in understanding the decay of radioactive isotopes in nuclear medicine and determining the shelf life of perishable goods.
Complex Calculations – Start Exploring Now!
Complex calculations refer to mathematical operations that involve intricate procedures or deal with complex numbers. These calculations often require a deep understanding of mathematical concepts and may involve multiple steps to arrive at a solution. Here’s more information on complex calculations:
Types of Complex Calculations:
Algebraic Equations: Solving algebraic equations involving variables, powers, and coefficients can be complex, especially when dealing with higher-order equations or systems of equations.
Trigonometric Functions: Calculations involving trigonometric functions such as sine, cosine, and tangent can become intricate, particularly when working with angles, trigonometric identities, and inverse trigonometric functions.
Calculus: Calculus deals with rates of change and accumulation, requiring complex calculations for differentiation, integration, and solving differential equations.
Linear Algebra: Involves operations with matrices, vectors, and linear transformations, often used in various fields including physics, engineering, and computer science.
Probability and Statistics: Calculating probabilities, expected values, and statistical measures like mean, median, and standard deviation can involve complex formulas and methods.
Applications of Complex Calculations:
Engineering and Physics: Complex calculations are essential in engineering disciplines such as structural analysis, fluid dynamics, and electrical circuit design. In physics, they are used in areas like quantum mechanics, relativity, and thermodynamics.
Finance: Financial analysts use complex calculations for risk assessment, portfolio optimization, option pricing, and modeling financial markets.
Computer Science: Complex calculations are employed in algorithms, data analysis, cryptography, artificial intelligence, and computer graphics.
Science and Research: Complex calculations play a vital role in scientific research across various domains including biology, chemistry, astronomy, and environmental science.
Medicine: From medical imaging to drug dosage calculations, complex calculations are indispensable in medical diagnostics, treatment planning, and pharmaceutical research.
Challenges and Considerations:
Precision and Accuracy: Complex calculations require careful attention to detail to ensure precision and accuracy, especially when dealing with large datasets or sensitive measurements.
Computational Resources: Some complex calculations may require significant computational resources, including high-performance computing systems or specialized software tools.
Interpretation and Validation: Interpreting the results of complex calculations and validating them against real-world observations or experimental data is crucial to ensure their reliability and relevance.
Signal Processing – Explore Cutting-Edge Solutions!
Signal processing is a crucial field in both engineering and mathematics, dealing with the manipulation, analysis, and interpretation of signals.
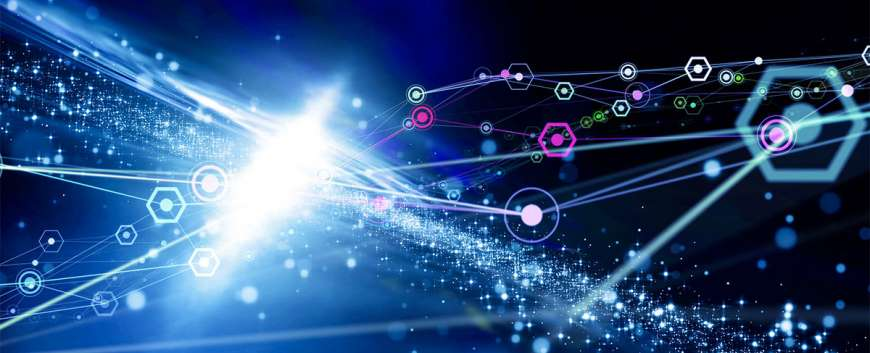
Signals, in this context, can represent any form of data, such as audio, video, images, or sensor readings. Signal processing techniques are applied in various industries, including telecommunications, audio processing, medical imaging, and more.
Basic Concepts:
Signals: Signals are representations of data that vary over time or space. They can be analog or digital and can take various forms, including continuous-time signals, discrete-time signals, and multidimensional signals like images.
Systems: Systems in signal processing are mathematical entities that transform input signals into output signals. They can be linear or nonlinear, time-invariant or time-varying, and causal or non-causal.
Time Domain and Frequency Domain: Signals can be analyzed in either the time domain or the frequency domain. Time domain analysis focuses on the behavior of signals over time, while frequency domain analysis decomposes signals into constituent frequencies using techniques like the Fourier Transform.
Applications:
Telecommunications: Signal processing plays a vital role in telecommunications systems for transmitting, receiving, and processing signals such as voice, data, and video. Techniques like modulation, demodulation, and error correction are employed to ensure efficient and reliable communication.
Audio and Speech Processing: In audio and speech processing, signal processing techniques are used for tasks such as speech recognition, audio compression, noise reduction, and equalization. Applications include voice assistants, audio streaming, and teleconferencing systems.
Medical Imaging: Signal processing is integral to medical imaging techniques such as MRI (Magnetic Resonance Imaging), CT (Computed Tomography), and ultrasound. It helps in image reconstruction, enhancement, and analysis, aiding in diagnosis and treatment planning.
Radar and Sonar Systems: Radar and sonar systems utilize signal processing for target detection, tracking, and imaging. Techniques like pulse compression, Doppler processing, and beamforming are employed to extract useful information from received signals.
Challenges and Future Directions:
Big Data and Real-time Processing: With the increasing volume and velocity of data, signal processing algorithms need to handle big data efficiently and perform real-time processing to meet the demands of modern applications.
Machine Learning and Deep Learning: Integrating machine learning and deep learning techniques with signal processing has led to advancements in areas such as signal denoising, classification, and prediction, opening up new possibilities for intelligent signal processing systems.
Privacy and Security: As sensitive information is often embedded in signals, ensuring privacy and security in signal processing applications is paramount. Techniques such as encryption, watermarking, and authentication are used to protect data integrity and confidentiality.
Algorithm Analysis – enhance your problem-solving skills!
Algorithm analysis is a crucial aspect of computer science and software engineering, focusing on evaluating the efficiency and performance of algorithms.
It involves assessing how algorithms behave in terms of time complexity, space complexity, and overall runtime performance. Let’s delve deeper into the key components of algorithm analysis:
Time Complexity:
Time complexity measures the amount of time an algorithm takes to complete as a function of the input size. It quantifies the number of operations an algorithm performs relative to the input size. Commonly expressed using Big O notation, time complexity helps us understand how an algorithm scales with larger inputs and enables us to compare the efficiency of different algorithms.
Space Complexity:
Space complexity evaluates the amount of memory space an algorithm requires relative to the input size. It considers both the auxiliary space (extra space used by the algorithm beyond input) and the space used by the input itself. Similar to time complexity, space complexity is often expressed using Big O notation and helps in analyzing memory usage and optimizing algorithms for efficient memory utilization.
Runtime Performance:
Runtime performance refers to how efficiently an algorithm executes in practice, taking into account factors such as hardware, programming language, and compiler optimizations. While time and space complexity provide theoretical insights, runtime performance offers practical measurements obtained through empirical analysis or benchmarking.
It helps in assessing real-world performance and making informed decisions when choosing algorithms for specific applications.
Complexity Classes:
Complexity classes categorize algorithms based on their time and space complexity characteristics. For example, algorithms with polynomial time complexity belong to the class P (Polynomial Time), while those with exponential time complexity belong to the class NP (Non-deterministic Polynomial Time). Understanding complexity classes allows us to classify and analyze algorithms based on their inherent computational properties and limitations.
Analysis Techniques:
Various techniques are employed to analyze algorithms, including mathematical analysis, empirical analysis, and experimental analysis. Mathematical analysis involves deriving theoretical bounds on time and space complexity using mathematical tools such as recurrence relations and asymptotic analysis.
Empirical analysis involves running experiments on actual implementations to measure performance under different conditions. Experimental analysis combines theoretical and empirical approaches to validate algorithmic predictions and refine performance estimates.
FAQ’s:
Q1: Can I expand logarithmic expressions with different bases?
Yes, you can expand logarithmic expressions with different bases using the same principles, but remember to apply the appropriate rules for each base.
Q2: Are there other rules for expanding logarithmic expressions?
Yes, apart from the product rule used in this article, there are also rules for quotient, power, and base change, among others.
Q3: Why is it important to understand logarithms?
Logarithms are crucial in various fields such as mathematics, engineering, finance, and computer science. They simplify complex calculations and are used in functions, algorithms, and formulas.
Conclusion:
In conclusion, expanding the logarithmic expression log20(mn5p) involves applying the product rule of logarithms, which states that the logarithm of a product is the sum of the logarithms of the individual terms. By breaking down the expression and treating constants separately, we can efficiently expand the logarithm to obtain its expanded form.
Understanding how to expand logarithmic expressions enables us to manipulate and analyze them more effectively, facilitating problem-solving and mathematical reasoning.